A step-by-step guide to OCR form processing
Optical Character Recognition (OCR) is a foundational technology that converts printed or typed text into machine-readable format. This capability has made it essential for form processing across industries. Different forms require different approaches - structured forms benefit from template-based OCR systems, while semi-structured and unstructured forms need more advanced extraction methods. In this blog, we will explore how OCR technology transforms paper forms into digital data, its core benefits, and how implementing it can streamline your workflow. Want to understand form processing with OCR? Let's dive into the details.
What's the difference between structured and semi-structured forms?
Every day, businesses process countless forms as part of their operations. These forms typically fall into two distinct categories:
i) Structured Forms ii) Semi-structured Forms
Understanding this distinction is crucial as it determines the processing approach. Let's examine each type:
Structured forms
Think of structured forms as having a rigid, predictable layout. Every field sits in exactly the same spot across all copies, with only the entered data changing. This consistency makes them ideal for OCR processing since the system knows precisely where to look for information.
While this fixed design enables accurate data extraction, challenges can arise. For instance, when text overlaps field boundaries or lines, OCR accuracy may suffer. A simple example: a "1" written too close to field lines might become invisible to the OCR engine.
Semi-structured forms
Semi-structured forms present a more complex challenge. In these documents, field positions can shift, and identifiers may appear in different locations. This variability creates problems for basic OCR tools, which might extract incorrect data due to inconsistent field placement.
To handle semi-structured forms effectively, OCR systems must employ sophisticated business rules that identify data points based on contextual clues rather than fixed positions. These rules look for patterns and relationships between elements to locate and extract the correct information.
Form processing: Use-cases
Let's explore how different industries leverage OCR for form processing:
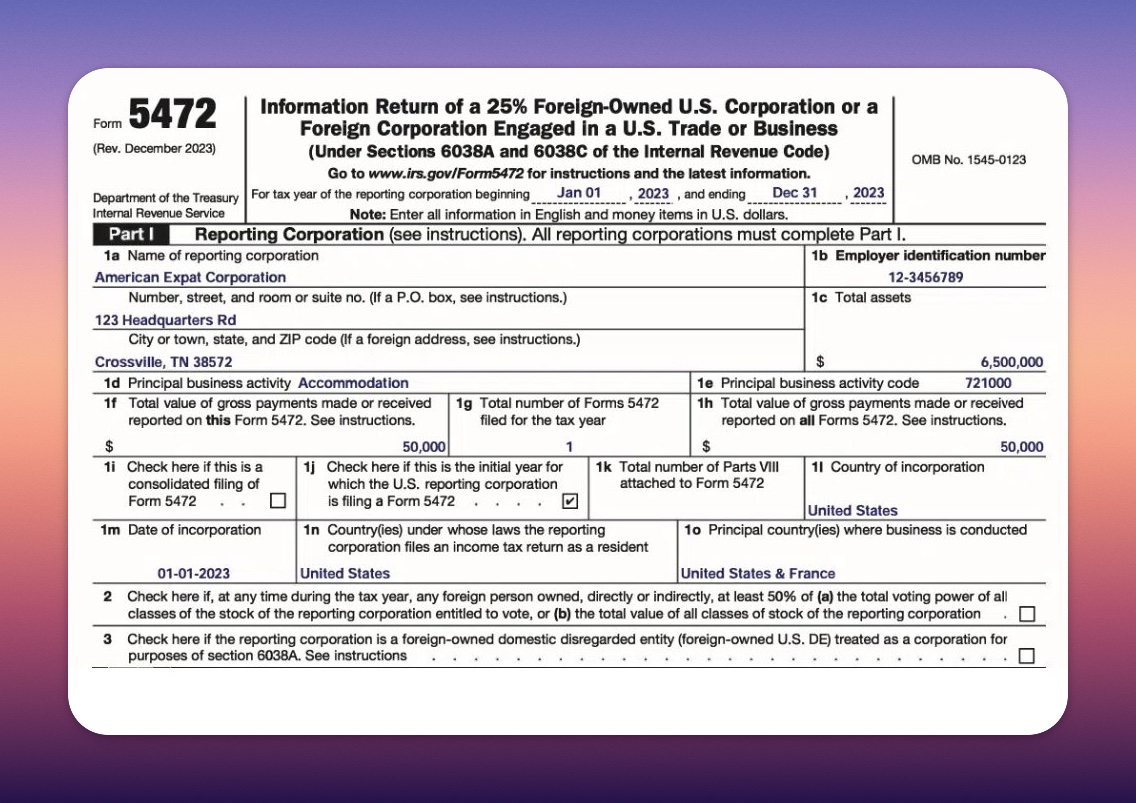
Tax Documentation
Tax forms represent one of the most common applications for OCR technology. These documents require precise data extraction for accurate processing. Key tax forms include:
- Form 1040 - Personal tax returns
- Form W-4 - Employee tax withholding
- Form W-9 - Taxpayer identification
- Form 941 - Quarterly employer returns
- Form W-2 - Annual wage reporting
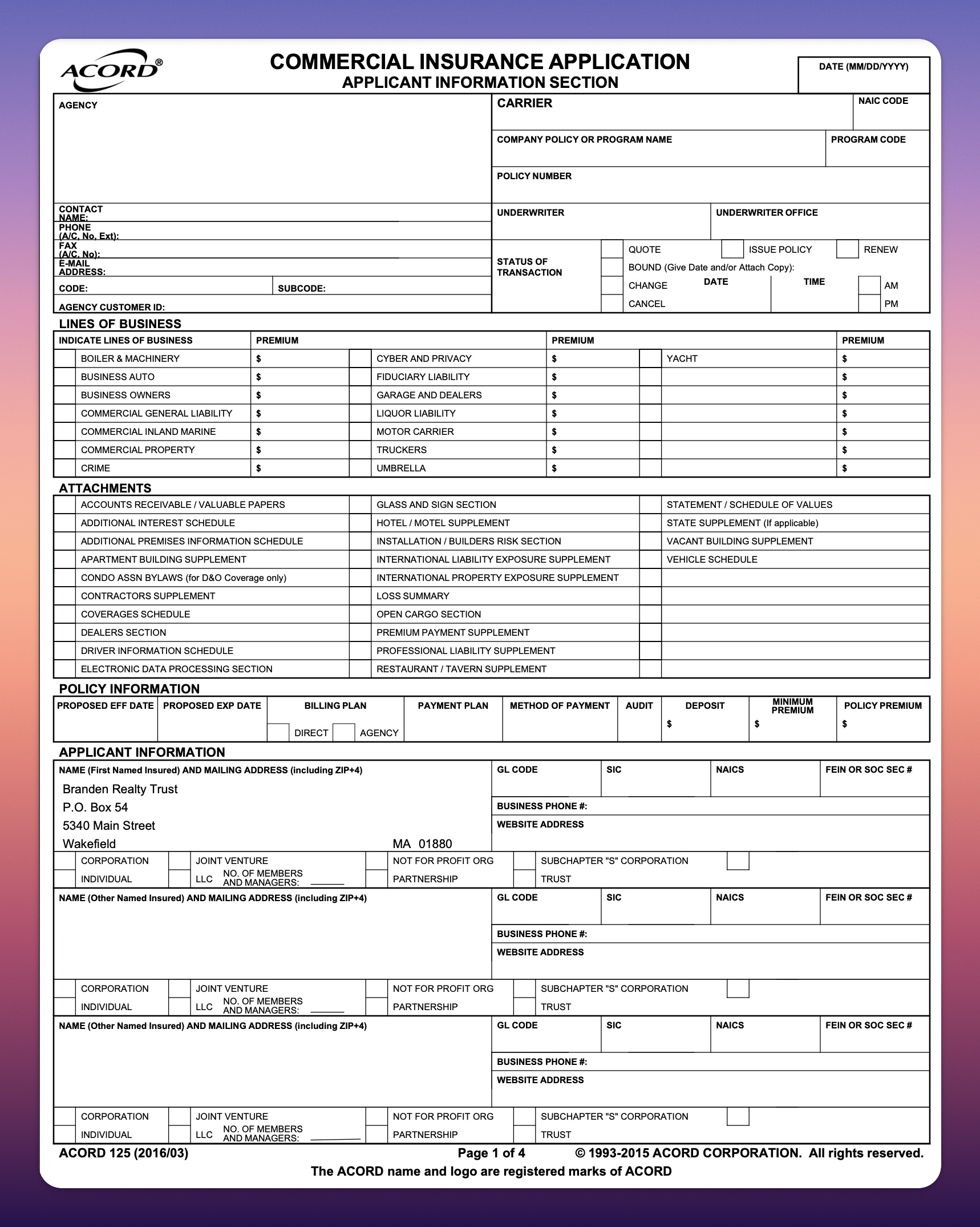
Insurance Documentation
Insurance companies rely heavily on standardized forms for policy management and claims processing. The industry standard ACORD forms come in multiple variations:
- ACORD 25 - Liability insurance certification
- ACORD 27 - Property insurance documentation
- ACORD 80 - Home insurance applications
- ACORD 90 - Vehicle insurance requests
- ACORD 125 - Commercial coverage applications
- ACORD 126 - General liability details
- ACORD 127 - Commercial auto information
Application Processing
Financial institutions and insurance providers handle numerous application forms daily. These documents contain crucial customer information needed for underwriting and assessment.
Modern OCR systems must first digitize paper forms before processing. Even PDF documents undergo conversion to image format to ensure consistent processing across all input types.
How does OCR form processing work?
The journey from paper to digital data involves several crucial steps:
Format detection
Initially, the system identifies the incoming document format. This step ensures all documents, regardless of their original format, convert to the appropriate image type for OCR processing.
Image pre-processing
Quality enhancement plays a vital role in successful OCR. The system removes visual noise - those unwanted variations in brightness and color that could interfere with text recognition. Advanced filtering techniques eliminate stray pixels and optimize image clarity.
Data Extraction
Let's examine the two primary extraction challenges:
Tables
Table extraction requires more than simple character recognition. OCR systems must understand structure and relationships between data points. The software analyzes lines, spacing, and visual patterns to accurately capture tabular data while maintaining its organizational context.
Key-Value Pair Mapping
Key-value pairs represent related data elements, like "Name: John Smith". Template-based OCR excels at extracting these pairs from structured forms where positions remain constant. However, semi-structured forms require additional intelligence to locate and associate related elements correctly.
Limitations of OCR form processing
Despite its capabilities, OCR technology faces several challenges:
- Font size variations - Extremely large or small text can pose recognition difficulties
- Directional constraints - Traditional OCR works best with horizontal text alignment
- Case sensitivity issues - OCR systems may struggle to differentiate between upper and lowercase characters in certain contexts
Intelligent Document Processing: The next evolution
Modern Intelligent Document Processing (IDP) systems overcome traditional OCR limitations through advanced AI capabilities. Key advantages include:
1. Scalability - Process higher volumes without sacrificing accuracy. IDP adapts to form variations automatically, eliminating template maintenance.
2. Efficiency - Automated processing frees staff from manual data entry, allowing focus on higher-value tasks.
3. Precision - Advanced IDP systems achieve over 99% field-level accuracy, with straight-through processing rates exceeding 95%.
4. Data Quality - Built-in validation rules and database cross-referencing ensure extracted data meets quality standards.
Ready to transform your form processing workflow? Consider implementing an OCR solution that matches your organization's specific needs and volume requirements.